ML and DL: Challenges
- Jessica Wang
- Jul 26, 2024
- 2 min read
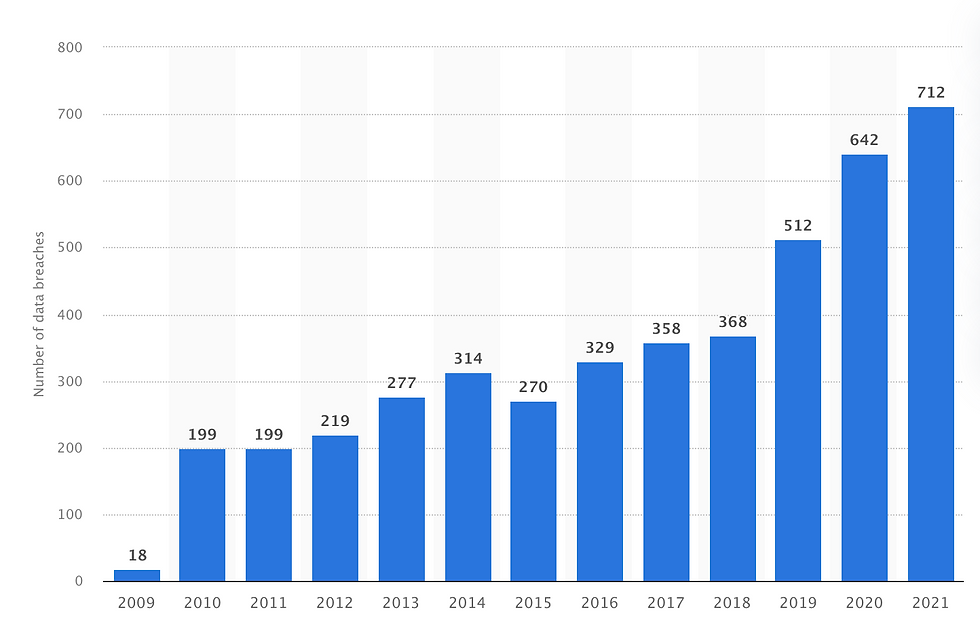
As ML (machine learning) and DL (deep learning) become more involved in healthcare, challenges become increasingly apparent. Cybersecurity poses a threat to nearly all medical devices that use ML and DL algorithms. For example, the large number of connected medical devices is always vulnerable to cyber threats. The FDA and European Union have acknowledged the need for guidelines regarding the cybersecurity of medical devices. Current guidelines already in place include HL7 (Health Level Seven International), which aids in securing the sharing of patient data through connected information systems. However, further implementation of guidelines and regulations is needed to improve overall healthcare even more.
There are also ethical concerns relating to ML and DL algorithms. Enforcing ML and DL in healthcare requires constant patient consent and privacy protection. There are also ethical issues regarding the bias and fairness of algorithms. Machine learning models learn from the data given to them. Considering this, if more data about a certain class is given to the model compared to a minority class, then less accurate predictions will be made for the minority class due to less data. This can cause bias and counteract the benefits of ML and DL. Transparency and responsibility are also sometimes overlooked. Healthcare professionals need to “come on one page” and unite on the basis of being responsible and transparent with technical support.
However, another challenge builds from the previous one. There are multiple cases where a lack of scientists and ML/DL engineers presents itself. This shortage results in an inadequate number of workers to support AI technically. It also makes it difficult to implement AI in the medical industry since there are fewer people to collect and clean data. The time it takes to clean up and verify unorganized data is consuming as well. If the data is not cleaned properly, ML and DL models can end up using incorrect data to learn and build machine learning projects.
References:
Maddali S. Various Challenges for Applying Machine Learning in Healthcare [Internet]. Medium. 2022. Available from: https://towardsdatascience.com/various-ways-at-which-machine-learning-could-be-used-in-medical-diagnosis-338c65393509
AI in Medical Devices and Healthcare: Challenges and What Lies Ahead | HTEC [Internet]. htecgroup.com. 2023. Available from: https://htecgroup.com/ai-in-medical-devices-and-healthcare-challenges-and-what-lies-ahead/
Machine Learning in Healthcare: Applications and Challenges - IFACET [Internet]. 2023. Available from: https://ifacet.iitk.ac.in/knowledge-hub/machine-learning/machine-learning-in-healthcare-applications-and-challenges/
Ellis RJ, Sander RM, Limon A. Twelve key challenges in medical machine learning and solutions. Intelligence-Based Medicine. 2022 Jun;100068.
Comments